Transforming Business with Data Annotation
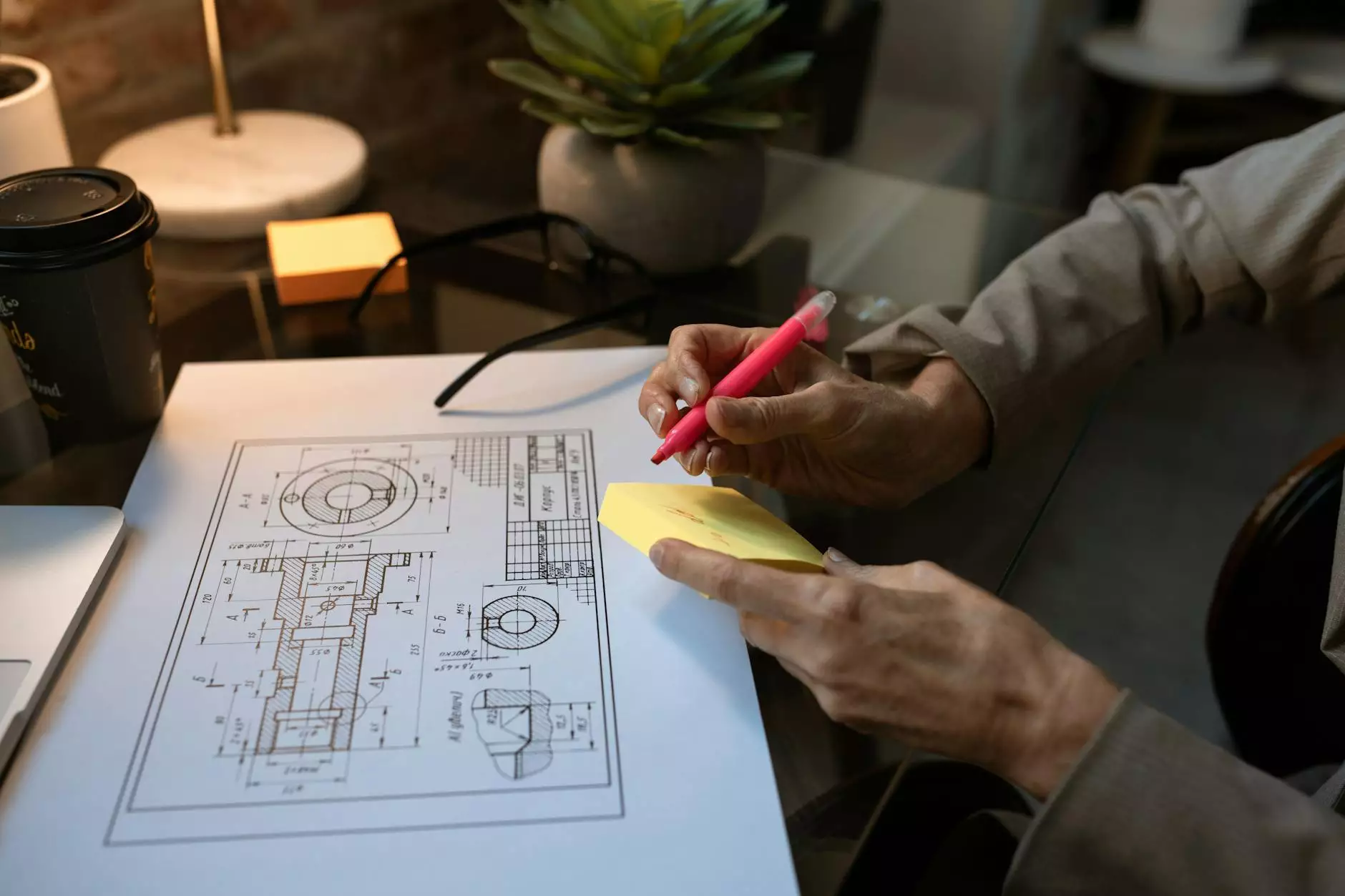
The business landscape has changed dramatically with the advent of artificial intelligence (AI) and machine learning (ML). Companies are increasingly leveraging data to enhance their operations, improve efficiency, and drive innovation. A crucial aspect of this data-centric evolution is data annotation, particularly in the context of visual data. In this article, we will explore the significance of the ability to annotate pictures, the tools available for businesses, and how these contributions can ultimately reshape your organization’s future.
Understanding Data Annotation: The Backbone of AI
At its core, data annotation is the process of labeling data to make it understandable for machines. This label helps algorithms learn and make predictions. For image-related AI applications, the ability to annotate pictures correctly is fundamental. Whether it’s for facial recognition, object detection, or image segmentation, these annotated datasets are what power training for machine learning models.
The Importance of Annotating Pictures
Why is annotating pictures so crucial in the realm of AI? Here are several key reasons:
- Enhanced Training Accuracy: Properly annotated images provide accurate data for machine learning models, leading to better performance and results.
- Rich Data Insights: Annotations can reveal underlying patterns and insights that can drive strategic decisions.
- Facilitating Automation: High-quality annotations enable businesses to implement automation solutions effectively, reducing human error and increasing efficiency.
- Improved User Experience: AI-powered applications that leverage annotated visuals often result in smoother and more intuitive user interactions.
Key Categories of Data Annotation
Data annotation can be categorized into various types based on the annotation methodology and application. Understanding these categories can help businesses decide which method suits their specific needs:
1. Image Annotation
This involves labeling images used for computer vision tasks, including:
- Bounding Box Annotation: Drawing rectangular boxes around objects within an image.
- Polygon Annotation: Defining the precise shape of an object; useful for detailed image segmentation.
- Landmark Annotation: Marking specific points on an object, such as facial features.
- Semantic Segmentation: Classifying each pixel of the image into object categories.
2. Video Annotation
Similar to image annotation but applied to video data, where frames are labeled to discern movements or objects over time.
3. Text Annotation
This method involves tagging and categorizing text data, which is crucial for natural language processing (NLP) applications.
4. Audio Annotation
Labeling sound and voice data to help train speech recognition models.
Choosing the Right Data Annotation Tool
When it comes to annotating pictures, having the right tools in your arsenal is vital. Here are some criteria to consider when selecting a data annotation tool:
- User-Friendly Interface: The tool should be easy to navigate and operate without extensive training.
- Collaboration Features: Tools that allow team collaboration can enhance efficiency and accuracy.
- Scalability: Consider whether the tool can handle a growing volume of data over time.
- Integration Capabilities: The ability to integrate with existing systems is essential for streamlined operations.
- Cost-Effectiveness: Evaluate the pricing model to ensure it aligns with your budget and expected ROI.
Best Practices for Effective Image Annotation
To maximize the benefits of annotating pictures, consider the following best practices:
1. Define Clear Guidelines
Establish detailed instructions for annotators so that everyone understands the criteria for labeling. This helps maintain consistency across the dataset.
2. Employ Quality Assurance Checks
Implement a review process to ensure that annotations are accurate. Regular audits can catch errors early and improve overall data quality.
3. Use Advanced Annotation Tools
Utilize sophisticated software that can facilitate the standardization of labels and support various annotation techniques.
4. Train Your Annotators
Invest time in training your data labelers to produce high-quality annotations. A well-trained team will be more efficient and precise in their work.
The Future of Data Annotation in Business
The demand for data annotation continues to rise as businesses increasingly rely on AI technologies. With every passing day, more sectors discover the potential of annotated data to revolutionize their operations, from retail and healthcare to agriculture and automotive industries.
As more organizations recognize the importance of visual data, the ability to annotate pictures becomes not only a benefit but a necessity. In the next few years, we can expect:
- Improved Automation: Further advancements in AI will make the process of data annotation more straightforward and automated.
- Regulations and Standards: As the use of AI grows, expect stricter guidelines and standards surrounding data annotation to ensure ethical practices.
- Increased Use of Synthetic Data: Combining synthetic data with real Annotated images will expand datasets and enhance training.
- Collaboration Between AI and Human Annotators: Hybrid models leveraging human insight and AI efficiency will become increasingly common.
Conclusion: Elevate Your Business with Data Annotation
In an era dominated by data, the ability to annotate pictures effectively holds the key to unlocking vast potentials within your business. By investing in quality data annotation tools and practices, organizations can enhance their AI applications, streamline operations, and gain valuable insights into their datasets.
The journey towards mastering data annotation can be transformative, paving the way for enhanced operational efficiencies and superior technological capabilities. As such, embracing this practice is not merely an option but a strategic imperative for businesses who aspire to thrive in the competitive landscape.