Unlocking the Power of a Machine Learning Labeling Tool for Business Success
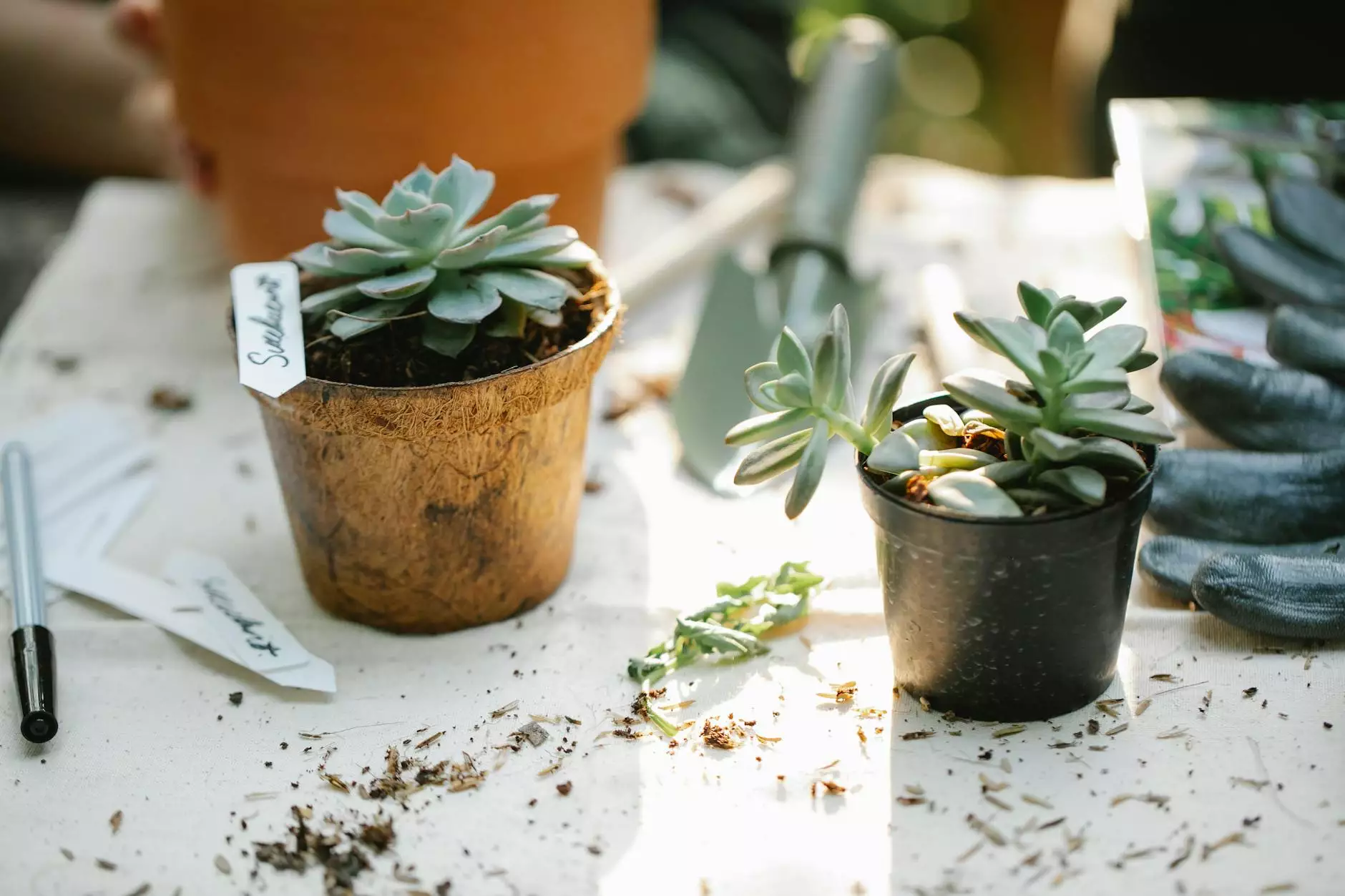
Understanding the Role of Machine Learning in Business
The rise of machine learning (ML) has transformed the landscape of modern business, offering innovative solutions for efficiency and productivity. By automating and enhancing decision-making processes, machine learning enables companies to leverage vast amounts of data, uncover insights, and drive strategic initiatives. At the core of successful machine learning applications lies one critical component: the machine learning labeling tool.
What is a Machine Learning Labeling Tool?
A machine learning labeling tool is software designed to assist in the data preprocessing phase of machine learning projects. Labeling data is crucial, as it allows algorithms to learn from examples and make predictions based on new, unseen data. Properly labeled data is the foundation for building robust models that can perform tasks such as image recognition, natural language processing, and autonomous driving.
The Importance of Data Labeling
Data labeling acts as the connective tissue between data and machine learning models. Without accurately labeled datasets, the performance of these models can significantly decline. Here's why labeling is so vital:
- Quality Training Data: High-quality, accurately labeled data ensures that machine learning models learn effectively, resulting in higher accuracy during inference.
- Cost Efficiency: Investing in proper data labeling upfront can save significant costs associated with model retraining and underperformance later.
- Improved Model Interpretability: Proper labels enhance the ability to interpret model decisions, leading to better trust and transparency.
How a Machine Learning Labeling Tool Works
Typically, a machine learning labeling tool works through a combination of manual and automated processes to create labeled datasets. Here's how it generally operates:
- Data Upload: Users upload raw datasets into the tool, which can be in various formats like images, text, or videos.
- Annotation Interface: The tool provides a user-friendly interface where annotators can label data according to specific guidelines.
- Quality Control: Many tools integrate quality control mechanisms to ensure annotations meet the required standards.
- Exporting Datasets: Once labeled, datasets can be exported in formats compatible with machine learning frameworks.
Benefits of Using a Machine Learning Labeling Tool
Implementing a machine learning labeling tool in your organization can yield numerous benefits:
1. Enhanced Efficiency
Automation features in many labeling tools can significantly speed up the process, allowing for rapid scaling of data preparation efforts. This means that businesses can focus on refining their models rather than getting bogged down in data preparation.
2. Cost-Effectiveness
By reducing manual labor and minimizing errors through automation, businesses can save costs associated with data labeling tasks. A well-chosen tool can provide a return on investment within a short time frame by accelerating the development process.
3. Improved Collaboration
Many modern labeling tools facilitate real-time collaboration, allowing teams to work together regardless of their physical location. This enhances communication and potentially leads to more innovative solutions.
4. Customization and Flexibility
Most machine learning labeling tools allow users to customize labeling workflows according to specific project needs. This flexibility means that businesses can adapt the tool to fit their unique requirements.
Choosing the Right Machine Learning Labeling Tool
When selecting a machine learning labeling tool, consider the following criteria:
- Type of Data Supported: Ensure the tool supports the specific types of data (images, text, audio, etc.) you are working with.
- User Experience: A user-friendly interface can significantly enhance productivity and reduce training time for new users.
- Integration Capabilities: Check if the tool can integrate seamlessly with your existing workflows or machine learning platforms.
- Cost: Evaluate pricing models and ensure it fits within your budget while still meeting your needs.
- Support and Community: Strong customer support and an active user community can be invaluable for troubleshooting and advice.
Integrating Machine Learning Labeling Tools into Your Software Development Process
Integrating a machine learning labeling tool into your software development process can transform how your teams approach data-driven projects. Here are steps to ensure successful integration:
1. Define Objectives
Before implementing a tool, clearly outline your objectives, such as improving data quality, reducing labeling time, or enhancing team collaboration.
2. Train Your Team
Provide comprehensive training for your team on how to use the labeling tool effectively. This ensures they are equipped to maximize the tool's potential.
3. Establish Labeling Guidelines
Define clear guidelines on how data should be labeled. This reduces inconsistencies and improves the quality of training data.
4. Monitor and Optimize
Regularly assess the performance of your labeling process and make necessary adjustments to improve efficiency and accuracy.
Real-World Applications of Machine Learning Labeling Tools
The application of machine learning labeling tools spans various industries, including:
1. Healthcare
In healthcare, labeling tools are crucial for annotating medical images, facilitating better diagnostic algorithms.
2. Autonomous Vehicles
For the automotive industry, labeling tools help create datasets for training self-driving cars to recognize different objects on the road.
3. E-commerce
E-commerce platforms utilize labeling tools to enhance product categorization and improve personalized recommendations.
4. Finance
In finance, machine learning tools can automate fraud detection by training models on labeled transaction data.
Future Trends in Machine Learning Labeling Tools
The future of machine learning labeling tools looks promising, with several trends shaping its development:
1. Increasing Automation
As AI technology advances, the automation of labeling processes will only increase, reducing the need for manual intervention and allowing teams to focus on higher-level tasks.
2. Enhanced AI-Assisted Labeling
Future tools will likely incorporate AI to suggest labels based on learned patterns, thereby increasing the speed and accuracy of the labeling process.
3. Collaborative Features
As remote work becomes the norm, collaborative features will play an increasingly vital role in facilitating teamwork on labeling tasks from different locations.
4. Cross-Platform Compatibility
Tools that can seamlessly integrate with various machine learning frameworks and programming environments will become necessary as businesses look to streamline their workflows.
Conclusion
The adoption of a machine learning labeling tool can position your organization to thrive in an increasingly data-driven world. With the right tool, companies can unlock efficiencies, enhance their data quality, and build more accurate predictive models. As machine learning continues to evolve, investing in robust labeling solutions becomes critical for any business aiming to stay ahead of the curve.
For businesses looking to leverage the full potential of machine learning, starting with an effective labeling strategy is essential. Options like Keymakr provide valuable resources and services to guide your journey in integrating machine learning into your software development processes.